Augmented fitness
Computational intelligence for weight lifting
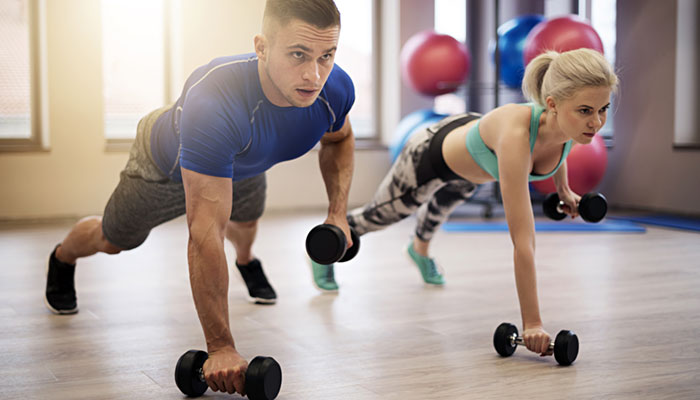
Project overview
Wearable fitness trackers, such as Fitbit, Garmin and Microsoft band, are becoming increasingly popular and inexpensive, coming in different shapes and sizes with different types of functionality. Though there are many fitness trackers available on the market today, these are mostly designed to support cardio-aerobic exercises with little qualitative feedback for weight training.
In this project, the research team is exploring how wearable technology can support weight lifters in analysing and improving their technique and performance. Incorrect technique is a major cause of injuries among weight lifters, with the potential for life-long impacts. By offering weight trainers a way to evaluate their performance, we can not only prevent injury, but also direct trainers into maximizing the effectiveness of their time at the gym. This is crucial for ensuring safe and optimal outcomes. The challenge, however, is in identifying the exercise a weight trainer is undertaking, and evaluating their performance of that exercise. To address this, we are designing novel methods to analyse human movements (eg: how a weight lifting exercise is executed in terms of the movement of the joints of the body) from motion sensors worn by the trainer. The outcome will be a guidance system that will warn, assist and advise trainers to maintain proper technique.
This project is also exploring how technology can support and motivate users at the gym. Given the worldwide obesity epidemic, motivating people to exercise remains a significant challenge with the potential to substantially improve their quality of life. Through this project, we will employ insights from fields such as gamification, collaborative systems and social psychology to design systems that motivate people to work out and maintain their exercise routine.
The planned outputs of this project will include novel machine learning techniques to identify, quantify and qualify weight training exercises, novel sensors for collecting exercise data and new visualisation techniques that will allow weight lifters and trainers to view and reflect over their performance.
Project team
- Eduardo Velloso, Lecturer, School of Computing and Information Systems, The University of Melbourne
- Yousef Kowsar, PhD Candidate, Microsoft Research Centre for SocialNUI, The University of Melbourne
- Masud Moshtaghi, Research Fellow, School of Computing and Information Systems, The University of Melbourne
- Lars Kulik, Professor, School of Computing and Information Systems, The University of Melbourne
- Chris Leckie, Professor, School of Computing and Information Systems, The University of Melbourne
- Eduardo Oliveira, Research Fellow, Melbourne Centre for the Study of Higher Education, The University of Melbourne
- Justin Fong, PhD Candidate, Mechanical Engineering, University of Melbourne
- Thuong Hoang, Lecturer, School of Information Technology, Deakin University
Contact details
Publications
Mirmomeni, M., Kowsar, Y., Kulik, L. & Bailey, J. (2018) An Automated Matrix Profile for Mining Consecutive Repeats in Time Series. In Geng X., Kang BH. (eds) PRICAI 2018: Trends in Artificial Intelligence. Lecture Notes in Computer Science, vol 11013. Springer, Cham. DOI: https://doi.org/10.1007/978-3-319-97310-4_22
Kowsar, Y., Moshtaghi, M., Velloso, E., Kulik, L., & Leckie, C. (2016) Detecting Unseen Anomalies in Weight Training Exercises. In Proceedings of the Annual Meeting of the Australian Special Interest Group for Computer Human Interaction (OzCHI 2016), November 2016, Launceston, Australia. [PDF]